Author | Jia Ruixue
Content Summary
In order to open the "black box" of the digital platform and explore the structural characteristics and risks of its personal data collection, the author takes the application platform (App form) that domestic users use more frequently in daily life as a sample, and uses the mobile phone permission mechanism and application platform privacy policy text as the data source. From the macro perspective of the "platform society", the content structure of personal data is characterized as three network models. Combined with social network analysis, it was found that the role of the "gatekeeper" of the Android mobile phone permission mechanism is weakening, and the presentation of privacy policies is difficult to ensure that users are fully informed, and the application platform is easy to realize the aggregation and use of personal data. According to structured theory, the content structure of personal data is the mediation and result of the continuous structure of personal data collection practices. This structure more reflects the "structural power" of platform enterprises, and there is an imbalance between the constraints of platform enterprises' data collection practices and user initiatives.
Keywords
Platform Social Personal Data Structured
Application Platform Privacy Policy
Text
1. Introduction
As a new digital medium, digital platforms have become a new scenario and new tool for people to carry out various practices, and have quickly extended to various fields such as clothing, food, housing, transportation, work, learning, and entertainment. "Platformization" is a special form or specific representation of "mediation" in new information technology and social environment, making "platform society" an accurate refinement of the current "mediated society". Platform society refers to a new form of information society in which humans carry out social practice activities and share social resources in various digital platforms under the new information technology environment, providing a conceptual basis and theoretical perspective for understanding the constructive role of digital platforms in the entire society. At the same time, the direct result of the platform migration of human production and life practices is that the digital platform, as a data-intensive infrastructure, records and processes user personal data at all times, and forms a content structure of personal data at the entire social level. In a platform society where data has become a new type of production factor and basic strategic resource, people enjoy the convenient and personalized service experience brought by data value-added, while hiding deep concerns about risks such as personal data leakage, abuse, illegal transactions, and privacy disclosure. As the primary link in data development and utilization, what structural characteristics and risks have been formed have become a more urgent issue at the moment.
People often use "black box" to describe a recording device, such as a data monitoring system, or a system with mysterious operating mechanisms. The digital platform obviously constitutes a black box that continuously collects user personal data, but its data collection mechanism is unknown to users. On the one hand, users are experiencing an omnipresent "sentimental harm" and privacy rights are difficult to protect their data rights; on the other hand, users lack the ability and are unwilling to invest time and energy to understand what personal data is collected for what purpose, and what structural characteristics does personal data form as a whole society. In order to make this black box intuitive, this study of jumped out of the micro perspective of a certain (category) digital platform, placed personal data collection in the macro perspective of the platform society where digital platforms are interrelated, and introduced the relevant theories from the structural perspective into personal data research: on the one hand, based on social network theory, networked representation of the content structure of personal data is conducted in a quantitative relationship model, and the structural characteristics and risks of personal data collection are explored; on the other hand, we learn from structured theory, reveal the structured connotation of personal data collection, and reflect on the relationship between the constraints of platform enterprise data collection practice and user initiative.
From focusing on the impact of media technology on audience attitudes, cognition and behavior to the materiality of digital media, the research scope of communication has been continuously expanded.As for the position of this research in the communication research context, the exploration of digital platforms and their data collection mechanisms can not only further broaden the theoretical orientation of digital media research, but also innovate the perspectives of privacy and personal data research, and the insight into the platform society will also help enrich the media and social research vision. At the same time, the practical significance of this study is to enhance people's understanding of the collection of their own data, provide innovative ideas for the governance of personal data from the platform's social perspective, and thus help digital platforms and data play an important role in social and economic operations, and prevent related risks.
2. Literature review and research questions
This study mainly reviews the literature from four aspects: platform and platform society, personal data and privacy, personal data collection mechanism, and structural perspectives, and then raises research questions.
(I) From platform to platform society
"Platform" as emerging technological, economic, political and cultural phenomena in the context of mediation has always been a hot topic of research in many disciplines: the field of engineering design focuses on the platform's "modular technical architecture"; the field of computer emphasizes that the platform is "reprogrammable"; the field of economics focuses on the "multilateral market nature" of the platform; the field of political economy criticizes "platform capitalism". As the communication academic community's understanding of "media" gradually transcends media in the sense of mass communication, "was not regarded as an object of media before...because of its digital connectivity, it became a media", the research on media has gradually jumped out of the text-centered thinking. Among them, platform research, as a new field of digital media research, has brought new issues and new perspectives to communication science, such as the digitalization, intermediary and availability of the platform as a medium, platform algorithms and platform labor, platform connectivity culture and dataization, etc.
Entering the 21st century, Castells' "network society" has always been an accurate summary of social forms under the influence of information technology. With the "platformization" of network infrastructure and the "infrastructureization" of digital platforms, digital platforms have integrated and developed with new information technology and become the material basis for human production and life practice, reorganizing and constructing various fields of society. The attention of the academic community has gradually shifted from the platform's user interface and functions to the user data integration of its backend database, from "platform as things" to "platformization as a process". Driven by the "platform paradigm", the online society has undergone platform transformation, and the platform society has thus been formed. Platform society is a collection of platformization at different levels, interpreting the inseparable relationship between digital platforms and social structures. Under this new context and theoretical perspective of media and social research, the imagination of platform research is further released. Therefore, we can no longer study a certain (category) platform in isolation. We urgently need to regard many platforms as interconnected networks and conduct overall research from the platform's social perspective. In addition, data collection is obviously not an individual action of some platforms, but a conventional practice of the entire society. This perspective also provides a more accurate context for the issue of data flow.
(II) From privacy to personal data
As platformization becomes increasingly deepening in social life, the liquidity and commercialization of data have become an inevitable real issue. As a category spanning the fields of computer, information management and law, "personal data" is both overlapping with "privacy" and bounded by each other: privacy emphasizes privacy, and its value is based on personal dignity and free development of personality, and the value of property is not prominent, and the protection of privacy rights is mainly passive defense; while personal data integrates personal interests and property interests, emphasizing the active use and control of individuals' own data during interpersonal communication, which has independent value and use value. Data utilization issues after "deidentification" or "anonymization" processing, as well as data asset issues associated with this constitute the main difference between the two.
For a long time, communication science has been processing the proposition of "personal data" within the scope of "privacy".With the development of information technology, privacy rights have evolved from the original "never-resident right" to the "information self-determination right". Even so, its standards and specifications are increasingly incompatible with the rapid development of digital economy , showing certain limitations. Especially in the digital platform has entered the "Illegal Rise 2.0" stage of the original accumulation of user data. In the platform society, users are conducting production activities of digital economy raw materials (data) for free, and everything is being digitized. Although public personal data that can identify natural persons and are not private and sensitive, is not protected by privacy rights, the personal data rights and interests of their data subjects should also be protected. my country has placed "privacy rights" and "personal information protection" under the Personal Rights Compilation of the " Civil Code ", clarifying the boundaries between the two. Therefore, at the moment when the logic of data is deeply rooted in social texture, the research focus of communication science urgently needs to shift from "privacy as secret" to "personal data as controlled", especially the empirical research on personal data from the perspective of platform research .
(III) Personal data collection mechanism
Personal data has both individual attributes, business attributes and public attributes. Its rights subjects are diverse and forms a complex power relationship and structure based on power, legal authorization or user authorization. In this power structure, platform companies must obtain the user's "informed consent" by formulating privacy policies and applying for device permissions to call the device before they can collect their personal data. Among them, the privacy policy is an important industry self-discipline measure for platform companies to eliminate user privacy concerns, and is also one of the basic qualifications for platform operations. It aims to help users understand what personal data the platform will collect, why it is collected, how it is collected, stored, used and protected, etc. The privacy policy is also regarded as a "bait" for the website to "tempt" netizens to actively provide their data and a disclaimer when infringement occurs. The permission mechanism is an access control mechanism built into the smart terminal operating system, which controls the application's access to system resources and personal data.
is the first level for the collection and use of personal data. The privacy policy and permission mechanism are generally presented and prompted in three ways: First, enhancement notification, when users download, install, register and log in, or use a digital platform for the first time, prompt and ask users for consent by default, manual checking, or non-selectable; second, instant prompt, in the process of using the specific services of the digital platform, pop up instantly in a prompt box and apply to the user for calls to the corresponding permissions; third, users actively query and manage. Even with privacy policies and permission mechanisms as guarantees, personal data collection still occurs in an asymmetric power relationship. Users have little choice as to whether the user is monitored, how the platform companies handle the collected data, and what actions will be taken against the user based on the conclusions drawn based on the data. In short, existing studies have mostly demonstrated the attributes of personal data rights, privacy policies and authority mechanisms from the jurisprudence level, but there is a lack of empirical research on basic issues such as "what functional requirements of digital platforms correspond to which personal data collection" and "what structural characteristics have been formed in the entire platform society".
(IV) Related theoretical basis of structural perspective
Things are composed of structures. The reason why a set of characteristics is called "structure" is because it has a stable decisive role compared to other characteristics. Different structural perspectives correspond to different theoretical foundations and analytical paths. This study is mainly based on the relationship tradition and cultural tradition of structural analysis. The relationship tradition mainly originates from the system theory and social network theory. In systems science, "structure" refers to the sum of the relationships and interactions between elements of the system. Once formed, it will in turn impose constraints and restrictive effects on the relationships and behaviors between elements. As an important form of structure, a network consists of nodes and connections, whose core is a collection of relationships, where nodes represent elements of the system, and connections between nodes represent interactions between elements. With the development and maturity of social network theory and social network analysis methods, using quantitative relationship models to network characterize social phenomena has become the mainstream research method.As an interface for the interaction between individual agency and structural constraints, social networks can simultaneously test actions and structures, and are one of the most powerful tools for structural analysis at present.
In terms of cultural tradition, the modern Western social theory community has such epistemological distinctions: various structuralism and functionalism that emphasize "structure", focusing on structure and constraint issues; various hermeneutic traditions of "individual", focusing on subjectivity, action and meaning. Giddens' structuring theory abandons the dualistic perspective that only studies society from the subject or object, and establishes a research idea for viewing society from the perspective of human "social practice". Its core can be refined into "duality of structure", and the actions in the structure and the structure in the action are dialectically integrated. "For the practice of repeated organization of the social system, the structural characteristics of the social system are both the intermediary of the latter and the result of its results." Through practice, the mutually constructed relationship between social structure constraints and individual behavior autonomy can be realized, and the structure can be produced and reproduced by humans with autonomous and active nature across time and space, and then reacted to human practice.
To sum up, this study will combine the structural perspectives of social network theory and structured theory. On the one hand, it will characterize the relationship structure between the personal data collector (application platform composed of different functional modules) and the collected content (personal data) through a network model, and discover the structural characteristics and risks of personal data collection; on the other hand, it will look at the compositional, practical and productive characteristics of the personal data content structure in a speculative way, and reflect on the interaction relationship between platform enterprises and users in the process of "structural-action" mutual construction.
(V) Propose of research questions
RQ 1: What types of mobile permission categories will the application platform collect user's personal data by applying for calling? What network structure does these application platforms form between the mobile permissions they will apply for calling?
RQ 2: What personal data will the application platform collect from users, and what network structure does these application platforms form between the personal data they collect?
RQ 3: What functional modules will the application platform collect user's personal data based on? What network structure will be formed between these functional modules and the personal data they need?
RQ 3a: Since it will be collected by the same functional module, what kind of network structure will be formed between personal data?
RQ 3b: Since the same category of personal data is required, what kind of network structure will be formed between functional modules?
RQ 4: How is the content structure of personal data formed?
3. Research and design
(I) Application platform sample selection
application platform and smart terminal platform represented by smartphones are digital platforms that domestic users use frequently in daily life. As of December 2021, there were 1.029 billion netizens in my country who accessed the Internet through mobile phones, accounting for 99.7% of the overall number of netizens. The number of apps monitored in the domestic market reached 2.52 million. In June 2020, the number of apps installed per person for 15-19 was the largest per person for mobile phones, with 83; the number of apps installed per person for mobile phones under 10 was the lowest, with 28. This study is based on the ranking of the number of active users of the entire network provided by the big data product "iReview Qianfan", and selected the top 80 in the May 2019 list as the research sample, covering five major application platforms: information reading, social interaction, e-commerce services, audio and video entertainment and practical tools.
(II) Coding and Data Collection
Since the mobile operating system, application platform, its privacy policies and permission mechanisms are always in a rapid iteration state, The encoding and data collection work of this study is mainly concentrated from July 8, 2019 to July 20, 2019. As a complex and comprehensive service, the application platform consists of multiple functional modules and multiple types of personal data. Therefore, this study first encodes the "functional module type" and "personal data category". See Table 1 for details of the category.Then, the two coders collected data on the privacy policy texts of 80 application platforms according to the encoding rules and recorded them in Excel in the form of "Application Platform-Functional Module-Personal Data" triple , with a total of 2197 records. Each line indicates that a certain application platform will collect a certain type of personal data for a specific functional module once. A total of 335 records (more than 5%) were randomly selected from 80 application platforms, and the independent coder test was tested, krippendorff's=96.7%, with high reliability. Finally, we check the permissions of the 80 application platforms installed in the mobile phone, collect the "Application Platform-Permissions" data and record it in Excel in the form of a binary group, with a total of 814 records. Each line indicates that a certain application platform will apply to call a certain type of mobile phone permission.
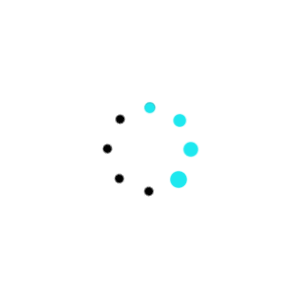
(III) Data processing and analysis
For the above collected data, this study mainly conducted an affiliate network analysis. membership network is a "2-mode network" used to represent the affiliation between a group of actors and a group of events. It consists of the actor set N={n1, n2,…, ng} and the event set M={m1, m2,…, mh}. It can be expressed as: the actor belongs to a certain event, or the actor is a member of a certain event. This study uses the "2-modular relationship matrix" to mark three affiliated networks, and the operation details are shown in Table 2. In addition, since the relationship between actors and events is duality - actors are associated with each other by the events to which they belong, and events are also associated with their actors, the affiliated network can be studied separately from the perspectives of actors and events. That is to say, the "2-modulo relationship matrix" can be converted into two "1-modulo relationship matrices": "co-participation matrix" XN records the number of events that are present for each pair of actors; the "event association matrix" XM records the number of actors with two events. This study will transform the above three "2-modulo relationship matrices" according to research needs. Finally, all the above relationship matrices were further analyzed through the social network analysis tool UCINET.
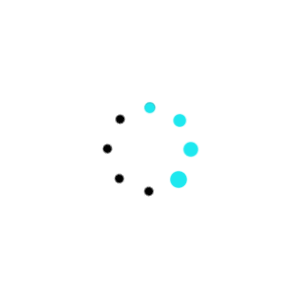
4. The study found that
constructs a network model, and this study analyzes the content structure of personal data from three levels and layers.
(I) Analysis of the application platform and the mobile permission category that it will apply for calls
For RQ 1, the "application platform-permission" composed of the Top 79 application platform belongs to the network topology model as shown in Figure 1. Among them, square nodes represent application platforms, with a total of 79; circular nodes represent mobile phone permissions that the application platform will apply for call, with a total of 21; a total of 814 connections are formed between the two types of nodes, with a network density of 0.5, indicating the ratio of the actual number of connections (814) to the theoretical maximum number of connections (79×21=1659), indicating that the two types of nodes are relatively closely connected. The node size represents the degree centering, that is, the number of other nodes directly connected to the node. The degree centering of the mobile phone permission node is equal to the number of application platforms to which it belongs. The average degree centering is 38.8, which means that each type of mobile phone permission will be applied for by 38.8 (49.1%) application platforms on average. As shown in Figure 2, almost all application platforms will apply for storage permissions, telephone (device information) permissions, location information permissions and camera permissions to call the phone. The degree centrality of the application platform node is equal to the number of permissions it will apply to call the mobile phone. The average degree centrality is 10.3, which means that each application platform will apply to call the mobile phone permissions on average. 360 Mobile Guardian (18), Application Bao (17), Tencent Mobile Manager (17), Baidu Mobile Assistant (17), 360 Mobile Assistant (17), QQ Synchronous Assistant (16), and other practical tool application platforms will apply for calling mobile phone permissions.
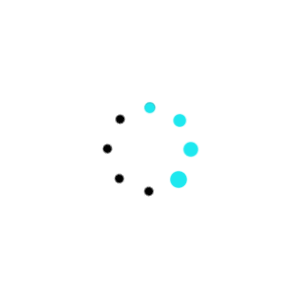
(II) Analysis of the category of personal data it will collect
For RQ 2, the "application platform-personal data" affiliated network topology model composed of the Top 80 application platforms is shown in Figure 3.Among them, square nodes represent application platforms, with a total of 80; circular nodes represent the categories of personal data collected by the application platform, with a total of 29; a total of 1,162 connections were formed between the two types of nodes, with a network density of 0.5. Each type of personal data is collected by 40 (50%) application platforms on average, among which operation and service log data (80), device data (80), location data (79), mobile phone number (75), network identity data (75), real name (68), and communication and interaction data (60) are the categories of personal data collected by most application platforms. Each application platform will collect 14.5 categories (50%) of personal data on average, among which Didi Chuxing (24), Ctrip (24), Gaode map (23), 360 Mobile Guard (22), Tmall (21), Kuwo Music (20), mobile Taobao (20), Industrial and Commercial Bank of China (20), etc. will collect more personal data, mostly e-commerce service application platforms.
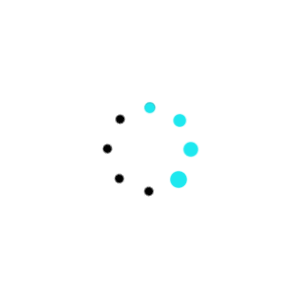
(III) Analysis of the type of functional modules of the application platform and the category of personal data of their requirements
1. "Functional Module-Personal Data" affiliated network analysis
For RQ 3, the "Functional Module-Personal Data" affiliated network topology model composed of the Top 80 application platform is shown in Figure 4. Among them, square nodes represent different functional module types of the application platform, with a total of 16; circular nodes represent the categories of personal data collected by the application platform to achieve different functional requirements, with a total of 29; a total of 251 connections were formed between the two types of nodes, with a network density of 0.5. Each type of personal data will be required by 8.7 categories (54.4%) functional modules, among which personal data such as device data (16), photo/video/record (15), location data (14), relationship data (12), network identity data (12), property data (11), operation and service log data (11), address (11), ID card (11), mobile phone number (11), real name (11), and other personal data will be required by more functional modules. Each type of functional module requires an average of 15.7 categories (54.1%) of personal data. Among them, there are many categories of personal data provided by third parties, such as services (21), travel services (20), financial services (20), customer service and after-sales service (20), operation and security guarantee (20), registration and login (19), other life services (19), commodity purchase (18), identity authentication (17) and other functional modules.
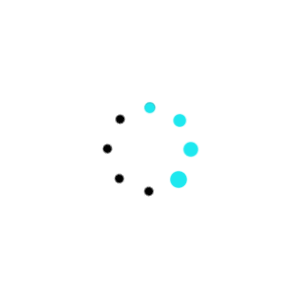
2. "Personal Data-Personal Data" 1-mode network analysis
For RQ 3a, the "Personal Data-Personal Data" 1-mode network converted from the "Functional Module-Personal Data" affiliated to the network has a total of 29 nodes and 391 connections. Since the joint participation relationship of personal data is "valued", the network density is equal to the average value of paired connections of personal data, which is 5.0, that is, each pair of personal data will participate in the construction of 5 types of functional modules on average. The degree centrality of a node indicates the number of times different categories of personal data will participate in the construction of functional modules. The average degree centering of personal data nodes in this network is 139.1, the maximum value is 235, and the minimum value is 42. Among them, personal data such as device data (235), photos/video/record (225), location data (205), relationship data (194), address (192), mobile phone number (192), real name (192), ID card (192), and other personal data participate in the construction of functional modules more often. Condensed subgroup analysis of the network can help us further understand which categories of personal data are more likely to gather together. Clique is a relatively strict concept of condensed subgroups, which is the largest complete subgroup that includes at least three nodes, and any two nodes are adjacent to each other and has a close relationship. For multi-value networks, the first thing to do is to determine the degree of cohesion of the subgroup, that is, the critical value c. The larger the value of c, the stronger the cohesion of the subgroup and the greater the relationship between nodes. The faction it contains is called the "C-level faction". There are 4 11-layer factions in the network (c=11), see Table 3. These factions are composed of all personal data categories that participate in the construction of at least 11 functional modules and overlap each other.
3. "Function Module-Function Module" 1-Mode Network Analysis
For RQ 3b, the "Function Module-Function Module" 1-Mode Network converted from "Function Module-Personal Data" belongs to the network has 16 nodes and 120 connections. It is a fully connected network, that is, any two functional modules will require the same type of personal data, and each pair of functional modules will require on average 9.5 types of personal data. The average value of the times different functional module types require the same type of personal data is 141.9, the maximum value is 187, and the minimum value is 70. Among them, the functional modules such as services (187), customer service and after-sales service (185), operation and security guarantee (183), travel services (175), financial services (175), other life services (166), and commodity purchases (164) provided by third parties have the same type of personal data as other functional modules. There are 4 15-layer factions in the network (c=15), see Table 3. These factions are composed of all functional modules that collect at least 15 types of personal data together and overlap each other.
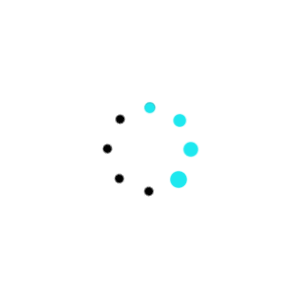
5. Research Conclusions and Discussions
This study mainly summarizes the structural characteristics and risks of personal data collection from the platform's social perspective from the role of the "gatekeeper" of the mobile phone permission mechanism, the presentation method of privacy policies, the aggregation and use of personal data and the secondary use of personal data, and draws on structured theory to reflect on the imbalance between platform enterprises and users.
(I) The role of the "gatekeeper" of the Android mobile phone permission mechanism is weakening
The operating system of the smartphone is the initial link that controls user data collection permissions, and the application platform is the direct carrier for collecting user data. The ideal running logic of the Android mobile phone permission mechanism is that the application platform needs to apply for system permissions in an explicitly declared manner (static way) in the AndroidManifest.xml configuration file, and in the code running stage, in the requested way (dynamic way). However, in actual operation, due to the openness of the Android system and the lack of application store security audits, application platforms can always use the inherent defects of the permission mechanism to collect user data beyond their functional requirements. is the "gatekeeper" for the application platform to collect user data, and the Android mobile phone permission mechanism has not fully played its role in protecting user personal data.
First, the application platform and the mobile phone permissions it will apply for invocation form a closely connected affiliate network. storage permissions, phone (device information) permissions, location information permissions, camera permissions, microphone permissions and address book (read contacts) permissions have become the "standard configurations" that every application platform will obtain, which means the general acquisition of personal data such as photos/video/records, file data, device data, location data and relational data. Second, it is not uncommon for application platforms to force and excessively obtain mobile phone permissions. For example, as long as the user disagrees, the application platform will continuously pop up the window to apply for permission. In order to have a smooth user experience, the user can only click to allow it, and once the permission is granted, the application platform will always have the permission. In addition, some application platforms require users to "package authorization" when installing. Third, the operation of the Android mobile phone permission mechanism depends too much on the user's judgment. In order to use the application platform normally, it is an undeniable thing for users to grant permissions to some extent. As for whether the personal data obtained through these permissions will be used for other purposes that are not related to the initial collection purpose, the user knows nothing. In response to the above situation, it is necessary to strengthen the privacy design concept of the operating system, and pre-embed intelligent programs related to data protection "embedded into the design standards of technology, business standards and physical infrastructure" to "make them the default rules for system operation" without the need for users to take too much action. At the same time, the application store must strictly review and remove applications that violate operating system permission requirements, and even be related to corporate credit, and jointly regulate the chaos in personal data collection from the source.
(II) The presentation method of the privacy policy is difficult to ensure that users are fully informed
Classification and grading are a perspective of understanding the world, a method of explaining things, and a basis for risk identification. It has become a consensus in the academic community to classify and classify personal data. Personal data categories can be divided according to the dimensions of use scenarios, security level, privacy level, sensitivity level, etc. However, only static and isolated content can be presented for personal data classification, while visualization of the relationship between personal data categories and application platform functional module types helps to characterize dynamic and related personal data content structure. In the privacy policy, the interrelated "functional module-personal data" has more governance-level operational significance than the current presentation method. The fine-grained presentation method of can simultaneously explain the categories and uses of personal data, which not only conforms to users' cognitive habits, but also alleviates the "information asymmetry" between users and platform companies to a certain extent. The nature of the ecosystem of the
platform determines that it will expand its functions in all aspects by accessing third-party services, and more functions often mean more data needs. We can summarize the personal data collected by the application platform into three categories: one is the data that must be collected to realize the basic functions of the application platform. If the user refuses, the platform cannot be used. For the supervision of this type of data, the Central Cyberspace Administration of Information Technology, the Ministry of Industry and Information Technology, the Ministry of Public Security, and the State Administration for Market Regulation jointly formulated the "Internet 5 for the scope of necessary personal information for the common types of mobile Internet applications in "; the second is the data collected to realize the additional functions of the application platform. If the user refuses, the expected effect of the service cannot be achieved; the third is the data collected and shared to realize the third-party services of the application platform. The third party abides by its own privacy policy. The platform can only urge it to provide sufficient security protection for user data and does not bear legal liability for its improper use. Based on this, the presentation of "functional module-personal data" can avoid putting users in the selection dilemma of "accepting all orders" or "negating all orders", allowing users to clearly recognize which personal data will be collected and what purpose they are used, and whether there are other alternatives, so as to make a more wise choice before using a certain function or service.
Although my country's laws stipulate that the processing of personal data should follow the "principles of legality, legitimacy, necessity and integrity" and "disclosed personal information processing rules, explicitly specifying the purpose, method and scope of processing". However, due to the formalization of the privacy policy, low readability, and non-compliance of the notification terms, there is a big gap between the "notification" of platform companies and the "information" of users. The "principle of informing consent" is therefore joked that informing is not real notification, and consent is not real consent. Compared with obscure and tedious policy texts, platform companies can actively inform users of the categories and purposes of collecting and using personal data based on visual methods, such as the "Functional Module-Personal Data" network, so that users can agree to authorize platform companies to process their personal data on the premise of full knowledge. In addition, the consent mechanism of the "package" and "option exit" model commonly adopted by platform companies makes personal data collection a "one-time deal" and it is difficult to ensure the effective consent of users. Therefore, classifies and grading personal data based on "functional module-personal data", and "builds a negotiable consent model of 'option exit' + 'option entry'", which will be conducive to the pre-risk control.
(III) The application platform is easy to realize the aggregation and use of personal data and the secondary use of
platform is the carrier of data connection. Compared with isolated and single data, only data in the network is valuable. The diversity of personal data needs of the same functional module in the application platform makes the aggregation and use of different categories of personal data a trend. The identity of the needs of different functional modules in the application platform for personal data makes it possible to use personal data secondary between different functional modules.Among them, "aggregation" refers to the calculation operation of creating new, higher-level data entities statistically defined by user characteristics or attributes defined by platform encoding.
The aggregation and use of personal data and the secondary use contain huge benefits and risks. For the former, connections are established between personal data that is simultaneously required by one or more functional modules. This potential connection indicates that these personal data are more easily aggregated, so that the application platform can achieve the integrated value of these data through diversified collection and aggregation use. However, due to the social and cultural context, aggregation establishes countless possibilities for re-numbering, recombining and recombining data. Therefore, platform companies should pay attention to the risks of re-identification and privacy disclosure brought about by personal data aggregation, carry out personal data security impact assessments, and take effective personal data protection measures based on risk assessment. For the latter, , a connection is established between functional modules of one or more types of personal data. This potential connection shows that due to the non-exclusiveness and non-lossiness of data, platform enterprises can only collect it once and perform "second-to-N times" based on the original data, thereby effectively reducing the cost of repeated collection and management of personal data, improving the efficiency of personal data use and fully tapping its value. However, platform companies should also pay attention to that the current principles of informed consent are often based on initial use. Before changing the purpose and method of personal data processing, the consent of the personal data subject should be obtained again to avoid putting users in the risk of out-of-control and "N use" black box.
(IV) The imbalance between the constraints of platform enterprise data collection practice and user initiative
With the help of the representation of network models, this study found that in the social perspective of interrelated platforms, unless the application platform services are not used, all personal data will eventually be handed over to the platform enterprises as a "consideration" for "free use": either through this type of functional modules, or through that type of functional modules; either through this type of application platform, or through that application platform. Moreover, personal data will be circulated at high speed and fully aggregated in the context of open and shared data. is based on multi-dimensional open relationships, and an open relationship network structure based on back-end personal data flow will be formed between application platforms, which will lead to problems such as expanding the risk range of personal data, increasing risks that individuals can identify and re-identify, and weakening individuals' control over their data. Among the personal data that is demanded by various functional modules, property data, bank cards, personal biometric data, whereabouts, health and physiological data, credit data, etc. are all sensitive personal data. "Once it is leaked or illegally used, it is easy to infringe on the personal dignity of natural persons or to endanger personal and property safety." Equipment data, operation and service log data, etc. are accompanying users' online interactions, that is, "machine-generated data" that does not contain user labor elements. Although it cannot be used to identify specific natural persons separately, it can often be combined with other data to identify specific natural persons. These aggregated data can even constitute a new type of privacy as an important source of user portraits such as personal interests, preferences, and behavioral habits. Moreover, mobile device identification codes such as International Mobile Device Identification (IMEI) and Media Access Control (MAC) addresses can uniquely identify a mobile device. Once the identification code is collected and bound to the user, the user's behavior on the device can be tracked.
The previous article has summarized the structural characteristics and risks of personal data collection and briefly proposed countermeasures. Next, this study will further reflect on the structured theory to answer RQ 4. The content structure of personal data is the mediation and result of the continuous structure of personal data collection practices. The main entities participating in this structured process are platform enterprises, users and government agencies, which play the role of personal data collectors, collectors and regulators respectively. The content structure of personal data is formed under the interaction of the three. Its structural characteristics indicate that the interests of the three are not balanced (this study mainly focuses on the first two), which more reflects the "structural power" of platform enterprises. In the process of mutual construction of "structure-action", platform enterprises are "structural occupants of rules and resources", and the balance of power is obviously biased towards it . It constructs the content structure of personal data through permission mechanisms, privacy policies, functional modules of the front-end user interface, automatic extraction and integration of back-end data, as well as powerful technical support such as cloud computing, big data, and artificial intelligence. The user's initiative is extremely limited. Faced with the data collection mechanism established by platform companies, "informed consent" seems to be behind the independent choice of users actually implies the suppression of the "structural power" of the platform companies, and the two are in an extremely unbalanced state. Although the aforementioned article mentioned that users can carefully use relevant functions and grant corresponding data based on fine-grained "functional module-personal data", the platform functions themselves are the result of careful design and manipulation. Research shows that: "Social media platforms are essentially data-based organizations that extract value and profits from their own social daily life." That is to say, even if users have a certain degree of initiative, it is difficult to exert too much influence on the overall architecture of the platform. Moreover, the current platform power is no longer limited to a single enterprise, but also lies in the coordination and rule-making power of a connected ecosystem as a whole, which reorganizes a series of relationships surrounding the platform. In addition, users' ability and willingness to reversely construct personal data content structure are also extremely limited. "Personal Data Literacy" includes the ability to identify data, data understanding, data reflection, data use and data tricks, which is obviously not something that any ordinary user can easily possess. Therefore, "the vast majority of individual actions have little impact on the structure... The mutual structure between social structure constraints and individual behavior autonomy is not an equal mutual structure." In other words, the data collection mechanism of the platform enterprise is far more restrictive to users than the user's initiative to this mechanism.
Digitalization brings humans into a stage of "deep mediation". In this media environment, all elements of the social world are closely related to digital media and their infrastructure. These software-based, highly interconnected digital media are no longer merely communication tools, but also serve as data generators, making automatic data processing a basic component of the construction of our social world. As the most typical digital medium with the most efficient value creation in our era, digital platforms are "making social life an open and extracted resource" and "reconstructing human life around maximizing profits in data collection." As humans become increasingly involved in "platform survival", personal data in all aspects will be aggregated into "data-based selves". In the "super panoramic prison" of platform society, individuals will become more and more transparent and present an objectification tendency. At the same time, the platform, a black box, will become increasingly secretive, especially the infrastructure platform that occupies the advantages of technology, information, market and other resources, will form strong "private power", so that "social and economic traffic will increasingly be regulated by a global online platform ecosystem driven by algorithms and data (mostly enterprises)." As a result, the commodified relationship between platform enterprises and users will form a new way of control. The entire society is like a "one-way mirror". "Important corporate actors have an unprecedented understanding of the details of our daily lives, and we know almost nothing about how they use this knowledge to influence us and the important decisions they make." "What we are afraid of is not the loss of privacy itself, but the one-way loss of privacy - that is, we cannot monitor those who monitor us." Over time, the personal data content structure that has stabilized in the spatial and temporal dimension will be continuously maintained and re-produced with the routine personal data collection practices, and the risks in it are also very likely to turn into crises. This study helps guide people to focus on the technological and material infrastructure that makes data collection and processing possible, and the political and economic relationships behind it.Faced with the imbalance between the constraints of platform enterprise data collection practices and user initiatives, it is particularly important to properly intervene in this structured process so that users' initiatives can be fully utilized to achieve "balance between individuals' interests in the protection of personal information, information operators' interests in the utilization of personal information, and the public interests of the state's management society." Improved or radical user empowerment plans formulated from the legal, economic, administrative and technical levels can be useful attempts to get out of structural dilemma.
6. Research limitations
First, this study encodes and collects the content about personal data collection in the application platform privacy policy text in the application platform privacy policy text in accordance with the "functional module-personal data". However, the privacy policies of some application platforms are not fully presented in this way. Moreover, the content in the privacy policy does not necessarily conform to actual practice. In the future, other methods and technical means to open the black box can be considered to further enrich the data source. Second, this study only selected the top 80 domestic apps as samples, and only examined the Android system operating environment. Although this case study is representative, it cannot fully reflect the complexity of the content structure of personal data from the platform's social perspective. In the future, we can consider further expanding the sample size and comparing the personal data collection situation of domestic and foreign platforms. Third, data classification and grading are an important topic in data governance. This study has conducted a typed analysis of personal data from the functional dimensions. In the future, further classification and grading should be combined with sensitivity and importance.
published "News and Communication Research" No. 7, 2022
Due to space, the public account will leave the comments. Please see the full version in the publication.
Edit | Zhu Jing