Many of the things we watch, read and buy enter our field of vision through recommendation systems of websites such as YouTube, Twitter and Amazon . The algorithm is personalized to obtain ad views, clicks, or purchases. Sometimes, the AI's advice frustrated us, as if they don't know us at all, or know us too much, predicting what will waste our time or get into the rabbit hole of anxiety and misinformation.
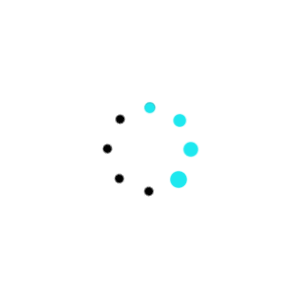
However, a more hidden dynamic may also be working. Recommendation System is not only likely to adjust to our most regrettable preferences, but actually shapes what we like, making the preferences even more regrettable. New research proposes a way to measure and reduce this manipulation. Recommended systems usually use an artificial intelligence called machine learning, which can discover patterns in the data, provide choices based on what we have done in the past, and guess what we will do now. A form of machine learning , called reinforcement learning (RL), allows artificial intelligence to play long-term games and make predictions a few steps ahead of time. This is the method DeepMind uses to defeat humans in Go and chess games.
If what we watch will affect what we like and people who like something (like cat videos) are more likely to continue watching (more cat videos), the recommendation system may recommend cat videos because it knows that this will pay off in the future. With RL, you have the motivation to change the board in order to win. The system will have the motivation to change human thinking to win the recommended game. The researchers first demonstrated how reinforcement learning can easily shift preferences. The first step is to recommenders establish a model of human preference by observing human behavior. To this end, they trained an neural network , an algorithm inspired by brain architecture. For the purpose of the research, they had the network build a single simulated user model, and they knew the actual preferences of this user so that they could more easily judge the accuracy of the model.
It watches the dummy make 10 consecutive choices, each with 10 options. It observes 1000 versions of this sequence and learns from each version. After training, it can successfully predict what the user will choose from in a series of past choices. Next, they tested whether a recommendation system could change the user's preferences after modeling it. In their simplified scheme, preferences are placed on a one-dimensional spectrum. This spectrum can represent political tendencies or dogs with cats or anything else. In the study, a person's preference is not a simple point on the line, but rather a distribution that indicates the possibility of choosing things in different areas of the spectrum. The researchers have specified two locations on the spectrum that are best for recommenders; perhaps those who like to click on these types of things will learn to like them more and keep clicking. The goal of the
recommender is to maximize long-term engagement. Here, the engagement of a given option is roughly measured by how well it matches the user's preference distribution at the time. Long-term engagement is the sum of the engagement levels of 10 consecutive options. A visionary recommender does not maximize engagement for each option in myopia, but rather maximizes engagement for the long term. As a potential side effect, it may sacrifice some early-stage sector engagement to drive users more satisfied in the next few rounds. Users and algorithms will learn from each other. The researchers trained a neural network to maximize long-term engagement. At the end of the 10 plate sequence, when it performed well, they reinforced some of its adjustable parameters. They found that this RL-based system does generate more participation than other trained systems.