Machine Heart Column
Machine Heart Editorial Department
From Researchers from Tsinghua University and Meta AI have proved the key to visual Transformer, namely input adaptive, long-range and High-order spatial interaction can also be used to convolutionize based on The framework is effectively implemented. The latest advances in
Visual Transformer show great success in various tasks driven by a new spatial modeling mechanism based on dot-generating self-attention. In this article, researchers from Tsinghua University and Meta AI have demonstrated that the key components behind visual Transformer, namely input adaptation, long-range and high-order spatial interactions, can also be effectively implemented through a convolution-based framework. The author proposes recursive gate convolution (
), which uses gate convolution and recursive design to perform high-order spatial interaction. The new operation is highly flexible and customizable, compatible with various variants of convolution and extends second-order interactions in self-attention to any order without introducing significant additional calculations.
can be used as a plug and play module to improve various visual Transformers and convolution-based models. Based on this operation, the author constructs a new universal visual backbone called HorNet. A large number of experiments in ImageNet classification, COCO object detection, and ADE20K semantic segmentation show that HorNet outperforms Swin Transformers and ConvNeXt with similar overall architecture and training configurations. HorNet also shows good scalability for more training data and larger model sizes. In addition to its effectiveness in visual encoders, the authors also show that
can be applied to task-specific decoders and continuously improve intensive prediction performance with less computational volume. The results of this paper show that
can be used as a new basic visual modeling module, effectively combining the advantages of visual Transformer and CNN.
- Paper address: https://arxiv.org/abs/2207.14284
- Code address: https://GitHub.com/raoyongming/HorNet
. Motivation
Convolutional neural network since AlexNet was introduced in the past decade ( CNN ) Significant progress has been made in deep learning and computational vision. CNN has many excellent features that make it naturally suitable for a wide range of visual applications. Translational equi-degeneration introduces useful inductive biases for the main visual tasks and enables transitiveness between different input resolutions. Highly optimized implementations make it very effective on high-performance GPUs and edge devices. The evolution of the architecture further increases its popularity in various visual tasks.
The emergence of a Transformer-based architecture greatly challenges CNN's dominance. By combining some successful designs in the CNN architecture with new self-attention mechanisms, Visual Transformer demonstrates leading performance on a variety of visual tasks such as image classification, object detection, semantic segmentation, and video comprehension. What makes visual Transformer more powerful than CNN? By learning new designs in Visual Transformer, some efforts have been made to improve the CNN architecture. However, the current work has not yet analyzed the effectiveness of dot-based self-attention in visual tasks from the perspective of higher-order spatial interactions.
Although complex and usually higher-order interactions exist between two spatial positions in the depth model due to nonlinearity, the success of self-attention and other dynamic networks suggests that explicit and higher-order spatial interactions introduced by structural design are beneficial to Improve the modeling ability of visual models. As shown in the figure above, ordinary convolution operations do not explicitly consider the spatial interaction between spatial locations (i.e., red features) and their adjacent areas (i.e., light gray areas). Enhanced convolution operations, such as dynamic convolution, introduce explicit spatial interactions by generating dynamic weights. The dot product self-attention operation in Transformers consists of two consecutive spatial interactions by performing matrix multiplication between queries, keys, and values. Trends in basic operations of visual modeling suggest that network capacity can be increased by increasing the order of spatial interactions.
In this article, the author summarizes the key factor behind the success of Visual Transformers is the new spatial modeling method of input adaptive, remote and high-order spatial interaction through self-attention operations. Although previous work has successfully migrated the meta-architecture, input adaptive weight generation strategies, and the large-scale modeling capabilities of visual Transformers to CNN models, higher-order spatial interaction mechanisms have not been studied. The authors show that using a convolution-based framework can effectively implement all three key elements. The author proposes recursive gate convolution (g nConv), which interacts with gate convolution and recursive designs at a higher order space. Unlike simply imitating successful designs in self-attention, g n Conv has several additional advantages: 1) efficiency. Convolution-based implementation avoids the quadratic complexity of self-attention. The design of incrementally increasing the channel width during the execution of spatial interactions also enables higher-order interactions with limited complexity; 2) scalable. Expand the second-order interaction in self-attention to any order to further improve modeling capabilities. Since there is no assumption on the type of spatial convolution,
is compatible with various kernel sizes and spatial hybrid strategies; 3) Translational equi-degeneration.
fully inherits the translational equi-degeneration of standard convolution, which introduces a beneficial inductive bias for the main vision.
Based on
, the author constructed a new universal visual trunk family called HorNet. The authors conducted extensive experiments on ImageNet classification, COCO object detection, and ADE20K semantic segmentation to verify the effectiveness of this model. With the same 7×7 convolution kernel/window and similar overall architecture and training configuration, HorNet outperforms Swin and ConvNeXt have great advantages on all tasks of varying complexity. The gap can be further expanded by using the global convolution kernel size. HorNet also shows good scalability, can be scaled to more training data and larger model sizes, reaching top-1 accuracy of 87.7% on ImageNet, 54.6% mIoU on ADE20K val, and COCO val The bounding box AP was pre-trained through ImageNet-22K. In addition to applying
in the visual encoder, the author further tested the universality of design on task-specific decoders. By adding
to the widely used feature fusion model FPN, the authors developed HorFPN to model higher-order spatial relationships of different hierarchical features. The authors observe that HorFPN can also continuously improve various intensive prediction models at lower computational costs. The results show that
is a promising visual modeling method that can effectively combine the advantages of visual Transofrmer and CNN.
. Method
.1 gnConv: Recursive Gated Convolutions
In this section, g n Conv, this is an effective operation to achieve long-term and high-order space interactions. g n Conv is constructed from standard convolution, linear projection, and element multiplication, but has input adaptive spatial mixing functions similar to self-attention.
Input-adaptive interactions with gated convolution
Visual Transformer Recent success mainly depends on the correct modeling of spatial interactions in visual data. Unlike CNNs that simply use static convolution kernels to aggregate adjacent features, Visual Transformer applies multi-head self-attention dynamic generation of weights to mix spatial tokens. However, the quadratic complexity largely hinders the application of visual Transformer, especially in downstream tasks, including the need for segmentation and detection of higher resolution feature maps. In this work, instead of reducing the complexity of self-attention as did previous methods, the authors seek a more efficient way to perform spatial interactions through simple operations such as convolution and fully connected layers.
The basic operation of this method is gate convolution (gConv).
is the input feature, and the output of gate convolution
can be written as:
where
,
is the linear projection layer that performs channel mixing, f is deep degree convolution.
, where
is a local window centered on i, and w represents the convolution weight of f. Therefore, the above formula clearly introduces the interaction between adjacent features
and
through element multiplication. The authors treat the interactions in gConv as first-order interactions, because each
interacts only once with its adjacent feature
.
High-order interactions with recursive gating
After achieving effective first-order space interaction with gConv, the author designed
html l0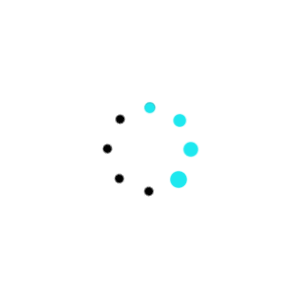
, a recursive gate convolution that further enhances the model capacity by introducing higher-order interactions. Formally, first use
to obtain a set of projection features
and
:
Then, the author recursively performs gating convolution by:
where, the output is scaled 1 /α to train stably.
is a set of deep convolutional layers,
is used to match dimension according to different orders.
Finally, the author feeds the output of the last recursive step
to the projection layer
to get the result of
. It can be easily seen from the recursive formula equation that the interactive order of
will increase by 1 after each step. Therefore, it can be seen that
realizes n-order spatial interactions. It is also worth noting that only a f is needed to perform the deep convolution to concatenate the feature
instead of calculating the convolution in each recursive step like in the equation above, which can further simplify the implementation and improve the efficiency of GPU. To ensure that higher-order interactions do not introduce too much computational overhead, the author sets the channel dimension in each order to:
This design shows that interactions are performed in a coarse to thin, where the lower orders are used with less channel calculation. In addition, the channel dimension of
is exactly 2C. Even if n increases, the total floating point can be strictly bounded.
where K is the kernel size of the deep convolution. Therefore,
implements higher-order interactions at a similar computational cost to the convolutional layer.
Long-term interactions with large kernel convolutions
Visual Transformer and traditional CNN are the receptive field. Traditional CNNs typically use 3×3 convolutions throughout the network, while visual Transformers calculate self-attention throughout the feature map or within relatively large local windows (e.g. 7×7). The receptive field in Visual Transformer can more easily capture long-term dependencies, which is also one of the key advantages of recognized Visual Transformer. Inspired by this design, there have been some recent efforts to introduce large kernel convolutions into CNNs. In order to enable
to capture long-term interactions, the author used two deep convolutions f implementations:
) 7×7 convolution. 7×7 is the default window/kernel size for Swin Transformers and ConvNext. [Study shows that kernel size has good performance on ImageNet classification and various downstream tasks. The authors follow this configuration, which is fairly compared with representative work of Visual Transformers and modern CNNs.
) Global Filter (GF).The GF layer multiplies frequency domain features with a learnable global filter, which is equivalent to a space domain convolution with global kernel size and circular padding. Modified versions of the GF layer are used by processing half channels using global filters and processing the other half channels using 3×3 depth convolution, and only later use the GF layer to preserve more local details.
Spatial interactions in vision models
The author reviewed some representative visual model designs from the perspective of spatial interaction. Specifically, the authors are interested in the interaction between feature x_i and its adjacent feature
. The key difference between Visual Transformer and previous architectures is that Visual Transformer has higher-order spatial interactions in each basic block. This result inspired the authors to explore an architecture that can achieve more efficient and efficient spatial interactions in more than two orders. As mentioned above, the author proposed that
can realize any order interaction with bounded complexity. It is also worth noting that, like other scale factors in depth models such as width and depth, simply increasing the order of spatial interactions without taking into account overall model capacity will not lead to a good trade-off. In this article, the authors work to develop a more powerful visual modeling architecture based on analyzing the spatial interaction order of carefully designed models. A deeper and formal discussion of higher-order spatial interactions may be an important direction in the future.
Relation to dot-product self-attention
Although the calculation of
in this article is very different from the dot product self-attention, But the author will prove that
also achieves the goal of input adaptive space mixing. Assume that M is an attention matrix obtained through multi-head self-attention (MHSA), write M as (
), because the mixed weights may vary in the channel. The spatial mixing result of the c-th channel at position i (before the final channel mixing projection) is:
where w_V is the weight of the V projection layer. Note that m_ij obtained by dot product operation contains first-order interactions. On the other hand, the output of
(before
) can be written as:
The following figure summarizes
Detailed implementation:
.2 Model Architectures
HorNet
can replace spatial hybrid layers in visual Transformer or modern CNNs. The author follows a meta-architecture with previous meta-architecture to build HorNet where the basic blocks contain spatial hybrid layers and feedforward networks (FFNs). According to the implementation of model size and depth convolution f_k, there are two model variant families named HorNet-T/S/B/L 7×7 and HorNet-T/S/B/L GF respectively. The authors regard the popular Swin Transformer and ConvNeXt as visual Transformer and CNN baselines, because the model in this paper is implemented based on a convolutional framework and has higher-order interactions like visual Transformer. For fair comparison with the baseline, the authors followed the Swin Transformers-S/B/L block count directly, but inserted an extra block in stage 2 to bring the overall complexity close to each stage of all model variations [2, 3, 18, 2] blocks are generated. Simply adjust the basic number of channel C to build models of different sizes and set the number of channels in 4 stages to [C, 2C, 4C, 8C] as convention. For HorNet-T/S/B/L, C=64, 96, 128, and 192 are used, respectively. By default, the interaction order of each stage (i.e. n in
) is set to 2,3,4,5, so that the channels of the coarsest C_0 are the same in different stages.
HorFPN
In addition to using
in the visual encoder, the author found that the
html in this article l3
can be an enhanced alternative to standard convolution that considers higher-order spatial interactions in various models based on convolution.Therefore,
replaces the spatial convolution in FPN for feature fusion to improve spatial interaction of downstream tasks. Specifically, the author added
after fusing the features of different pyramid levels. For object detection, the author replaces the 3×3 convolution after the top-down path with , at each level. For semantic segmentation, the author simply replaced the 3×3 convolution after the multi-order feature map concatenation with
, because the final result is directly predicted from this concatenated feature. The authors also provide two implementations, called HorFPN 7×7 and HorFPN GF, determined by the choice of f_k.
. Experiment
ImageNet The classification experiment results are summarized in the above table. The model in this paper achieves very competitive performance through state-of-the-art visual Transformers and CNNs. It is worth noting that HorNet goes beyond Swin Transformers and ConvNeXt, which have similar overall architecture and training configurations across various model sizes and settings.
The author evaluated the HorNet semantic segmentation task on the ADE20K dataset using the commonly used UperNet framework. All models are trained with 160k iterations using the AdamW optimizer, and the global batch processing size is 16. The image size during training is 512 × 512 for the pretrained model of ImagNet-1k (HorNet-T/S/B) and 640 × 640 for the ImageNet-22K pretrained model (HorNet-L). The results are summarized in the left part of the above table, where single-scale (SS) and multi-scale (MS) mIoUs on the validation set are reported. The authors also evaluated the model for this article on the COCO dataset. The author uses the cascading Mask R-CNN framework to use the HorNet-T/S/B/L backbone for object detection and instance segmentation. Following Swin and ConvNeXt, the authors used a 3× schedule with multi-scale training. The right part of the above table compares the box AP and mask AP of the HorNet model and the Swin/ConvNeXt model in this article.
The author now presents another application of the proposed
, that is, as a better fusion module, it can better capture higher-order intersections between different level features in intensive prediction tasks Mutual. Specifically, the authors directly modified FPNs for semantic segmentation and object detection, such as SuperNet and Mask R-CNN, respectively. The results are shown in the table above, where the authors compare the performance of HorFPN and standard FPN in this article on different backbones, including ResNet-50/101, Swin-S, and HorNet-S 7×7. For semantic segmentation, the authors found that HorFPN can significantly reduce FLOPs (∼50%) while achieving better mIoU. The table above shows the ablation experiment results of this method.
The above figure shows the trade-off comparison between Swin, ConvNeXt and HorNet.
. Summary
The author proposes recursive gate convolution (
), which is effective and scalable with gate convolution and recursive design. High-order spatial interaction of shifting and changing. In various visual Transformers and convolution-based models,
can be used as a replacement for spatial mixing layers. On this basis, the authors constructed a new universal visual backbone HorNet family. A large number of experiments have proved the effectiveness of
and HorNet on commonly used visual recognition benchmarks.
Finally, the author has summarized the HorNet network code in the following Github library, with the address:
https://github.com/xmu-xiaoma666/External-Attention-pytor ch
This library is a core code of top-level papers for novice Library . It summarizes many core codes of top-sum papers, including Attention, Self-Attention, Backbone, MLP, Conv, etc.
. HorNet combined with YOLOv5 model application
YOLOAir library has applied HorNet network to the YOLO model, and the following three methods are combined with the YOLOv5 model:
. Example of using gnconv module in YOLOv5
. Example of using HorBlock module in YOLOv5
- . Example of using HorNet backbone network in YOLOv5
Due to limited space, the specific code and methods can be obtained in the following GitHub library:
YOLO target detection library for scientific research novices: https://github .com/iscyy/yoloair
reference Link:
https://arxiv.org/abs/2207.14284
https://github.com/raoyongmin g/HorNet
https://github.com/xmu-xiaoma666/External-Attention-pytorch
https://github.com/iscyy/yoloair