Recently, Stanford University released the latest DAWNBenchmark results. In terms of the total training time of image recognition (ResNet50-on-ImageNet, above 93% accuracy), Huawei Cloud ModelArts ranked first, taking only 10 minutes and 28 seconds, an increase of nearly 44% from the second place. Results prove that Huawei Cloud ModelArts has achieved a lower cost, faster and more extreme experience.
Stanford University DWANBench is an international authoritative benchmark test platform used to measure the training and inference performance of end-to-end deep learning model. The corresponding rankings reflect the current leading deep learning platform technology in the global industry. Computation time and cost are key resources for building deep models. DAWNBench provides a common set of deep learning evaluation metrics for evaluating training time, training costs, inference latency, and inference costs on different optimization strategies, model architectures, software frameworks, cloud and hardware.
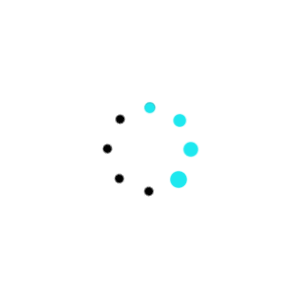
Stanford University DAWNBenchmark latest results
As one of the most important basic technologies of artificial intelligence, deep learning has gradually extended to more application scenarios in recent years. As deep learning models become larger and larger, the amount of data required increases, the training and inference performance of deep learning will be the top priority. Huawei Cloud ModelArts will combine Huawei's full-stack advantages in AI chips, hardware, cloud facilities, software and algorithm to create a faster and affordable AI development platform.
will be analyzed in depth below, how Huawei Cloud ModelArts achieves the ultimate performance - 128 GPUs, ImageNet training time 10 minutes.
1. Deep learning has been widely used, with models increasing, data growing, and the demand for accelerated training in deep learning is increasing
In recent years, deep learning has been widely used in computer vision , speech recognition, natural language processing, video analysis and other fields, and can serve video surveillance, autonomous driving, search recommendation, dialogue robots and other scenarios, and has broad commercial value.
In order to achieve higher accuracy, the amount of data and models required for deep learning are usually very large, and training is very time-consuming. For example, in computer vision, if we train a ResNet-50 model with 1 V100 GPU on the ImageNet[1] dataset, it will take nearly 1 week. This seriously hinders the development progress of deep learning applications. Therefore, the acceleration of deep learning training has always been an important issue that concerns the academic and industrial circles, and is also a pain point for the main application of deep learning. Fast.ai, led by several professors such as
Jeremy Howard, is currently focusing on deep learning acceleration, and the shortest time to train the ResNet-50 model with 128 V100 GPUs on the ImageNet dataset is 18 minutes.
However, the recent emergence of BigGAN, NASNet, BERT and other models indicates that training models with better accuracy requires more powerful computing resources. It can be foreseen that in the future, as the model increases and the amount of data increases, the acceleration of deep learning training will become more important. Only by having end-to-end full stack optimization capabilities can the training performance of deep learning be maximized.
[1] The ImageNet data set referred to in the article contains 1,000 categories, with a total of 1.28 million pictures. It is the most commonly used and classic image classification data set and a subset of the original ImageNet data.
2. Huawei Cloud ModelArts set a new record, with the "extreme" training speed
Huawei Cloud ModelArts is a one-stop AI development platform that has served the AI model development of major product lines within Huawei company . Over the past few years, it has accumulated multi-faceted optimization experience such as cross-scenarios, soft and hard collaboration, and end-cloud integration. ModelArts provides multiple modular services such as automatic learning, data management, development management, training management, model management, inference service management, and market, so that users at different levels can quickly develop their own AI models.
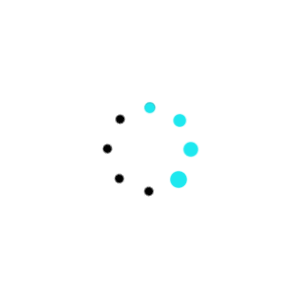
Figure 1. Huawei Cloud ModelArts Function View
In the model training section, ModelArts achieves training acceleration through coordinated optimization of hardware, software and algorithms. Especially in deep learning model training, Huawei abstracts the distributed acceleration layer and forms a general framework - MoXing (the pinyin of "model" means that all optimizations revolve around the model).Using the same hardware, model and training data as fast.ai, ModelArts can shorten the training time to 10 minutes, creating new records and saving users 44% of their time.
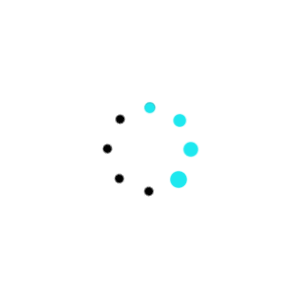
Figure 2. Training speed based on MoXing and ModelArts is improved
3. Distributed acceleration framework MoXing
MoXing is a distributed training acceleration framework developed by the Huawei Cloud ModelArts team. It is built on the open source deep learning engines TensorFlow, MXNet, PyTorch, and Keras, making these computing engines more distributed and more ease of use.
high-performance
MoXing has built-in multiple model parameter slicing and aggregation strategies, distributed SGD optimization algorithm, cascading hybrid parallel technology, hyperparameter automatic tuning algorithm, and optimized in many aspects such as distributed training data slicing strategies, data reading and preprocessing, distributed communication, etc. Combined with Huawei Cloud Atlas high-performance server, it realizes the acceleration of distributed deep learning collaborative optimization of hardware, software and algorithms.
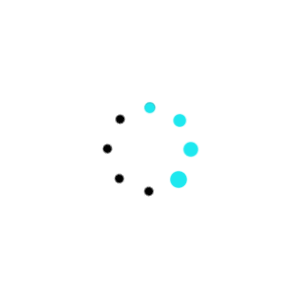
Figure 3. Huawei Cloud MoXing Architecture Diagram
Easy to use: Let developers focus on business models and worry about other
In terms of ease of use, upper-level developers only need to pay attention to business models, no need to pay attention to lower-level distributed APIs, only input data, models and corresponding optimizers based on actual business definitions. The training script has nothing to do with the running environment (standalone or distributed). The upper-level business code and distributed training engine can be completely decoupled.
4. From two major indicators, MoXing's key technologies for distributed acceleration
. When measuring the acceleration performance of distributed deep learning, there are two important indicators as follows:
1) throughput, that is, the amount of data processed in unit time;
2) convergence time, that is, the time required to achieve a certain convergence accuracy.
throughput generally depends on optimizations in server hardware (such as AI acceleration chips with more and greater FLOPS processing capabilities, larger communication bandwidth, etc.), data reading and caching, data preprocessing, model calculation (such as convolution algorithm selection, etc.), communication topology, etc. In addition to low-bit computing and gradient (or parameter) compression, most technologies will not affect the model accuracy while improving throughput. In order to achieve the shortest convergence time, it is necessary to optimize the throughput while also adjusting parameters. If the parameter adjustment is not good, the throughput is sometimes difficult to optimize. For example, when the ultra-parameter batch size is not large enough, the parallelism of model training will be poor, and the throughput will be difficult to increase by increasing the number of calculation nodes.
The ultimate indicator for users is convergence time, so MoXing and ModelArts implement full-stack optimization, greatly shortening the training convergence time. In terms of data reading and preprocessing, MoXing uses multi-stage concurrent input pipelines to make data IO not a bottleneck; in terms of model calculation, MoXing provides mixed precision calculations composed of half-precision and single-precision for the upper layer model, and reduces the losses caused by accuracy calculation through adaptive scale scaling; in terms of hyperparameter optimization, dynamic hyperparameter strategies (such as momentum, batch size, etc.) are used to minimize the number of epochs required for model convergence; in terms of underlying optimization, MoXing combines the underlying Huawei self-developed server and communication computing library to further improve distributed acceleration.
5. Comparison of test results, speaking with data
is generally trained on the ImageNet dataset. When the Top-5 accuracy is ≥93% or the Top-1 accuracy is ≥75%, the model can be considered to be converged.
The model training convergence curve we tested is shown in the figure below. Here, the Top-1 and Top-5 accuracy are the accuracy on the training set. In order to achieve the ultimate training speed, additional processes are used to verify the model during the training process. The final verification accuracy is shown in Table 1 (including comparison with fast.ai). The model corresponding to Figure 4(a) has a Top-1 accuracy of ≥75% on the verification set, and the training time is 10 minutes and 06 seconds; the model corresponding to Figure 4(b) has a Top-5 accuracy of ≥93% on the verification set, and the training time is 10 minutes and 58 seconds.
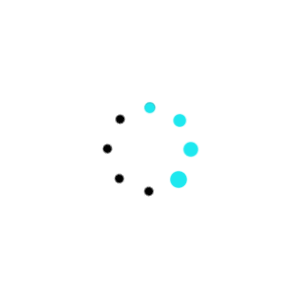
Figure 4. ResNet50 on ImageNet training convergence curve (the accuracy on the curve is the accuracy on the training set)
Table 1. Comparison of training results between MoXing and fast.ai
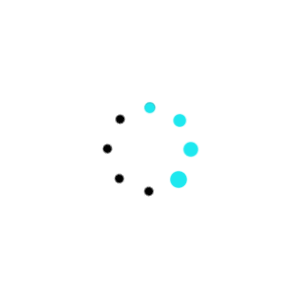
6. Future prospects - Faster AI development platform
Huawei Cloud ModelArts is committed to providing users with faster AI development experience, especially in model training. The built-in MoXing framework has greatly improved the training speed of deep learning models. As mentioned earlier, deep learning acceleration is the result of a multi-faceted collaborative optimization of distributed training frameworks and optimization algorithms from the underlying hardware to the upper-level computing engine, and then to the higher-level. Only with full-stack optimization capabilities can users' training costs be minimized.
Following, Huawei Cloud ModelArts will further integrate the advantages of hardware and software integration, providing a deep learning training platform that is full-stack optimization from chips (Ascend), servers (Atlas Server), computing communication library (CANN) to deep learning engines (MindSpore) and distributed optimization framework (MoXing). In addition, ModelArts will gradually integrate more data labeling tools to expand its application scope, and will continue to serve smart cities, intelligent manufacturing, autonomous driving and other emerging business scenarios, and provide users with more inclusive AI services on the public cloud.
Currently, Huawei Cloud ModelArts is in public beta. Everyone is welcome to try it out. For details, please refer to Huawei Cloud official website ModelArts.
----------------------------------------------------------------------------------------------------------------------------------------------------------------------------------------------------------------------------------------------------------
2. This article is for readers' reference only. This website has not verified the content and does not guarantee its originality, authenticity, completeness and timeliness.