Until today, how many problems remain unresolved in the research and implementation of medical imaging AI? The complexity and diversity of
tasks, the non-standard isolation of data, sparse and noisy annotations, and the fragility and instability of models have become unavoidable problems for medical imaging AI scholars. In the real environment, AI models face more complex tests and greater uncertainties.
2020 can be called the "first year of medical AI commercialization". A number of medical AI products have received official approval. While people are marveling at the “speed of China’s AI” and before the large-scale commercialization of medical imaging AI, we still need to think calmly about the current issues.
On January 9, 2021, the Zhongguancun Medical Artificial Intelligence Seminar was held.
This seminar was co-sponsored by the "Chinese Journal of Image and Graphics" and the Medical Artificial Intelligence Branch of the Chinese Society of Biomedical Engineering. Professor Tian Jie of the Institute of Automation, Chinese Academy of Sciences, Professor Gong Qiyong, Vice President of West China Hospital, and Institute of Computing, Chinese Academy of Sciences Professor Zhou Shaohua and associate researcher Zhao Di from the Institute of Computing Technology of the Chinese Academy of Sciences shared the latest research and application progress in medical imaging.
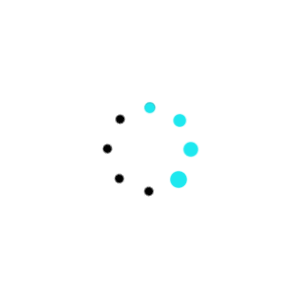
Professor Zhou Shaohua is a researcher at the Institute of Computing Technology, Chinese Academy of Sciences, and a part-time professor at Chinese University of Hong Kong (Shenzhen).
has won the Oscar for Invention, Siemens Inventor of the Year, University of Maryland ECE Distinguished Alumni, etc. He serves as the treasurer and director of the MICCAI Association. He has served as the co-chairman of the MICCAI 2020 program and the field chairperson of AAAI, CVPR, ICCV, MICCAI and NeurIPS conferences. .
Lei Feng.com learned that at the end of 2020, Professor Zhou Shaohua was selected as a Fellow of the National Academy of Inventors (NAI). NAI is a non-governmental, not-for-profit membership organization founded in 2000. NAI Fellow is the highest honor awarded by the academy to academic innovative inventors. It aims to recognize academic innovative inventors who have a significant impact on the quality of human life, economic development and social well-being.
has 1,403 Fellows so far (including this year’s new Fellows), including 38 Nobel Prize winners, 63 U.S. National Medal of Technology and Innovation, and U.S. National Medal of Science. Science) recipients, 556 academicians of the National Academy of Sciences (NAS), the National Academy of Engineering (NAE) and the National Academy of Medicine (NAM), 137 presidents of American research universities or heads of research institutions, etc.
These academicians from all over the world hold more than 42,700 US patents, created 36 million jobs and more than 2.2 trillion US dollars in revenue. In his speech at
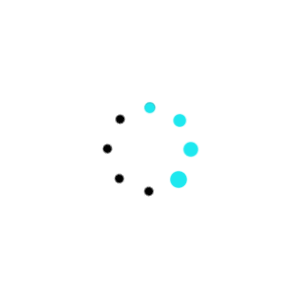
, Professor Zhou Shaohua listed seven major problems currently faced by medical imaging AI. Moreover, he shared his latest research ideas and application progress around deep learning automation , general representation learning, learning and knowledge fusion and other technologies.
With the consent of Professor Zhou Shaohua, we provide PPT for everyone to study and download. Follow the public account "Medical and Health AI Nuggets" , and reply " Zhou Shaohua " in the dialog box to obtain it.
The following is the content of Professor Zhou Shaohua’s speech. Lei Feng.com has modified it without changing the original meaning.
Zhou Shaohua: Thank you very much for the invitation to the conference. I will share the characteristics, technology and trends of medical imaging AI analysis from the perspective of algorithm . To conduct medical image analysis and processing, you must first know the characteristics of medical images that are different from natural images. Below, I will introduce its characteristics from the seven elements of image, data, disease, annotation, sample, task, and security.
First of all, medical imaging is multi-mode and high-definition. Common imaging multimodal modalities include X-ray, CT, MRI, PET-CT, and ultrasound. Moreover, the image accuracy of a single modality (such as CT) is now very high. Once the accuracy is high, we can use existing rendering technology to render the image as if it were taken by a camera. Of course, this also brings certain challenges to GPU training. The second characteristic of
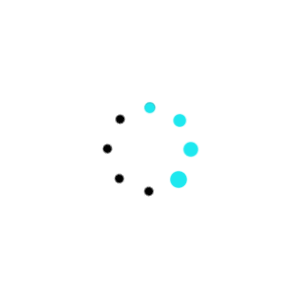
is that the data is non-standard and isolated. There is no uniform standard for medical imaging data collection, and different hospitals and imaging departments have different collection protocols. Moreover, imaging data are not interoperable between different hospitals and belong to an isolated island state. Even the data between different departments in the same hospital are not interoperable.
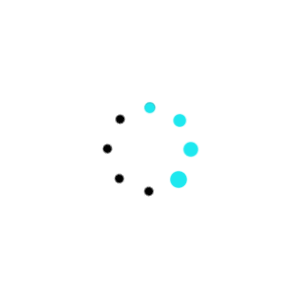
Furthermore, medical images are representations of disease.
Kahn’s Radiology Gamuts is a disease knowledge base that defines approximately 17,000 entries. Each item can be imagined as a related concept in the report, which requires a large knowledge base to support it at the bottom. Therefore, it is extremely complex to build a complete imaging diagnosis system.
Moreover, relatively common diseases such as pulmonary nodules occupy a large amount of data; on the contrary, a large number of diseases only have very little data, and the entire distribution presents a typical long-tail distribution. In addition, for emergencies like COVID-19, data collection is very difficult from the beginning. In short, the long tail of the disease is sudden.
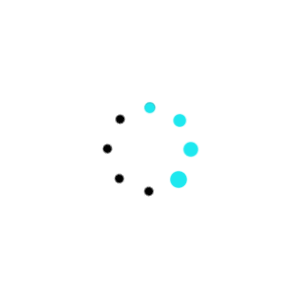
From the annotation level, data annotation is also relatively sparse. I extracted some annotation numbers provided by the organizers of the 2019 MICCAI competition, some of which were only 33 cases; there were also seemingly large-scale data sets (320,000 cases), but they did use a 64×64 patch as a sample.
Of course, the industry has also made a lot of efforts to launch some large data sets.
Additionally, even with labeled data, the annotations are often noisy. (i) As can be seen from the above figure, there are obvious differences in the labeling of organs by different doctors. (ii) Using this image report as the gold standard and extracting annotation information from it is also problematic.
statistics show that 15% of the report content does not completely accurately describe the image information. Even if the same image is viewed by two different doctors, 30% of the content may be inconsistent, which fully proves that there will be a lot of noise in the annotation. Therefore, the annotations are sparse and noisy.
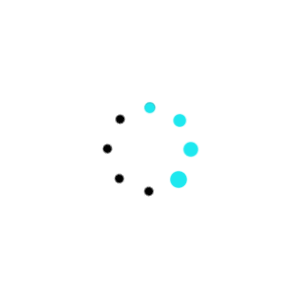
assumes that the annotation is sufficient and there is no noise. In practice, we face the problem of different samples and uneven .
For example, the problem of classifying benign and malignant pulmonary nodules. The left is a positive sample and the right is a negative sample. It can be seen that even within the same category, the morphological differences are very large. Judging from the proportion of samples, the number of negative samples is much larger than that of positive samples, several orders of magnitude higher. In addition, many negative samples look very much like positive samples.
These also bring many difficulties to us using machine learning for medical image analysis.
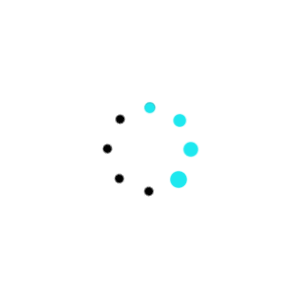
From a task level, if we want to build a very large AI system, we can look at how many tasks there are in total.
demonstrates several typical tasks here, including finding feature points in skull X-rays, brain registration based on different modalities, detecting tumors based on mammography, segmenting multiple abdominal organs, and simulating coronary blood flow. . These are five different missions.
Recall that medical imaging has different modalities, different disease types, and different technologies. If you arrange and combine these elements, you will find that tasks are complex and diverse .
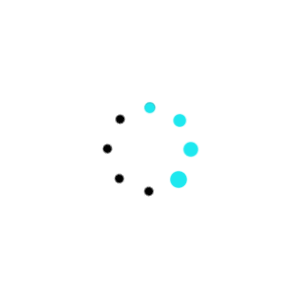
The other one is the security of medical imaging. Compared with natural images, medical images are more fragile and unstable, that is, their security is fragile and unstable. The left side of
is the X-ray of the skull just shown. We designed an algorithm for feature point detection. These green feature points are the locations we want detected by the algorithm. However, if a little interference is added to the image, the positions of these feature points can be manipulated arbitrarily.
In this example, we can manipulate these feature points into the shape of a letter "M", and our human eyes cannot detect the changes in the image.
Therefore, this algorithm is in a very fragile state. Adding some changes that are not easy to pay attention to the original image will have a huge impact on the output results.
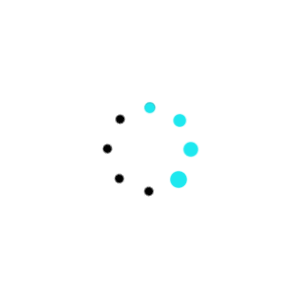
We also conducted some quantitative research. Suppose an attack with intensity less than one gray level is performed on a medical image with the intention of changing the output result. The goal of our attack is to reduce or increase the average value of the neural network features as much as possible.
One of the images shown above is a fundus image and the other is a natural image. It can be seen that the changes between the two are very different. After medical images are disturbed, the feature values can easily be reduced by more than 50%, while the changes in natural images are relatively weak. As the number of layers in the network deepens, this phenomenon further intensifies and becomes more and more unstable.This also proves from the side that medical imaging is a relatively unstable state and is easily affected.
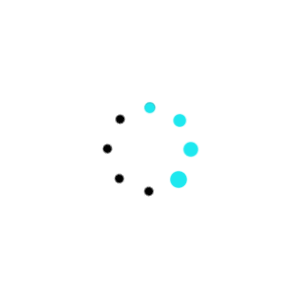
Considering these characteristics of medical imaging, can we design algorithms specifically?
Currently, the hottest algorithm is training deep neural networks, so-called deep learning. The assumption of this algorithm is: we have a single task and a large amount of labeled data, that is, "small task, big data". Under this condition, the current deep neural network can achieve very good results.
For example, many companies can truly reach practical levels in a certain type of single-task imaging products. However, this model is not easily scalable and cannot build a comprehensive system that meets all tasks of radiologists.
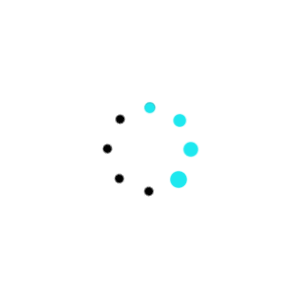
The actual situation requires us to solve the problem of "large tasks, small data ", that is, there are a large number of complex and diverse tasks, and each task has a small amount of annotated data. This poses a new challenge to algorithm researchers: can we design some new algorithms to achieve better results.
"Big tasks, small data" is a very broad concept, and different categories of trending technologies have emerged in different directions. Today I will mainly introduce three types of technologies: deep learning automation, general representation learning, and the integration of learning and knowledge .
deep learning automation
The concept of deep learning automation is relatively easy to understand.
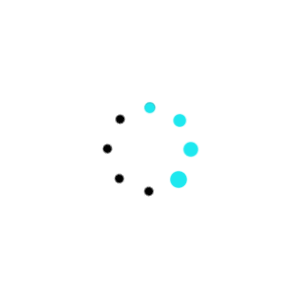
This is a very simple framework.
Assume that there is an input image X, an output variable Y, and a neural network f is learned in the middle, and its parameter is W. We will assume that there is a bunch of training data, which is {(Xi, Yi)}, and then construct an optimization problem and define some loss functions or regular terms to learn W.
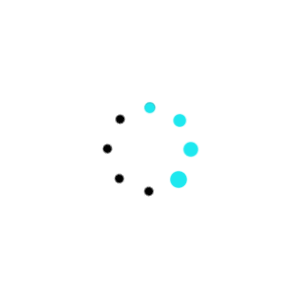
In this framework, there are actually many artificial parts (as shown by the yellow mark in the picture).
The first one is that a lot of Yi needs to be annotated, and the larger the amount of annotated data, the better. Therefore, our first research is whether we can find some efficient labeling algorithms (such as self-supervised, semi-supervised, weakly supervised learning, etc.) to reduce the demand for labeling.
Secondly, the learning process itself is an optimization process. There will be an objective function. So we have to propose such a loss function and regular terms, which are also artificially defined. Now, some studies have also proposed to make the objective function more clearly consistent with the problem raised through learning methods.
In addition, it is a problem of network structure. Many current practices are to directly train an existing neural network without carefully adjusting the structure. Therefore, we need to study whether there is a structure that is most suitable for this problem specifically. Current methods include network structure search and meta-learning. Another point of
that is easily overlooked is the expression aspect. Because X has given its expression, but Y can introduce different expressions. Different expressions are also important, because they will affect the training itself. The main reason is that when calculating the gradient return, its size is different; and the ease of gradient return is the most important indicator when training a neural network. Therefore, we hope to find a good expression of Y that can better implement gradient return.
output expression
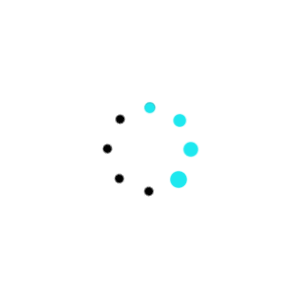
Let’s first look at an expression example: designing a universal tumor detection solution. Generally for this kind of detection problem, we will use Bounding Box (BBox). The two-dimensional BBox has four parameters, namely center point, length and width. You could also train a neural network to find boxes, but this box representation is very inefficient with gradient backpropagation. Because it controls the training of a neural network that may contain millions of parameters by the difference of four parameters, it is not very efficient.
Based on this, we proposed the concept of Bounding Map (BMap), turning a box that was originally four parameters into an image-like expression. The advantage of this is that each pixel can return a guiding gradient information. Therefore, the gradient information is richer and the neural network will learn better.
We made a comparison of three commonly used methods of box expression.After using our new expression, the performance has been greatly improved.
self-supervision
Next, we will introduce the concept of self-supervision.
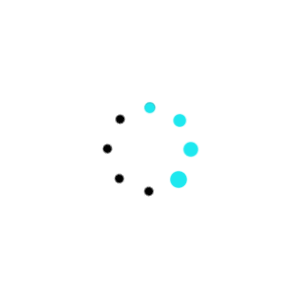
In practice, we may only have a small amount of labeled data, but a large amount of unlabeled data. Therefore, an intuitive idea is: can we use these unlabeled data to help the training of target tasks or target models. This is the starting point of self-supervision.
What we have to do is to define a proxy task (proxy task), which generates supervision signals. Using this supervision signal, we can train a neural network and obtain a pre-trained model. Because we have a large amount of unlabeled data, and generally the larger the amount of data seen in neural network training, the more robust the representations it learns will be. The design of
proxy tasks has become a research topic. We can design different agent tasks. If designed well, very good network representations can be learned.
Next, we use a small amount of labeled data of the target task to apply the pre-trained model to the transfer learning method to obtain the final target model.
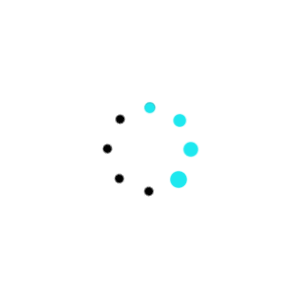
We have also done some exploration in this aspect.
We have defined a "Rubik's Cube Restoration" task. You can imagine dividing a three-dimensional image into 8 blocks (2×2×2). During training, you can scramble it like a Rubik's Cube, but the scrambling process of any image is known, and we can restore the Rubik's Cube by training the neural network.
During the restoration process, the neural network learns the representation of the image itself, and then transfers it to the target task. Above is our final result. Compared with the method of training from scratch using only labeled data, the improvement of our self-supervised method in tasks such as stroke classification and brain tumor segmentation is obvious. Of course, the premise is that we have a small amount of labeled data and a large amount of unlabeled data.
Obviously, the effects of the agent task and the target task are related. At present, many fellow scholars have tried to propose different agent tasks. We explored another possibility, which is not to propose new agent tasks, but to integrate existing agent tasks to see if the results will be better.
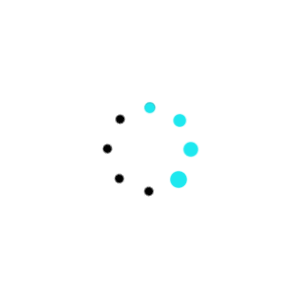
Our intuition is also very simple: after training, each agent task should find a part of the feature space, and the target task is likely to occupy another part of the feature space. This effect will be better if the proxy task completely covers the feature space desired by the target task.
Therefore, if the feature space similarity obtained by each agent task is smaller and the complementarity is stronger, after fusion, the space they cover will be larger, and the greater the help to the target task will be.
We designed an algorithm based on this to find these complementary agent tasks. In the figure above, we find that out of the six different agent tasks, three of them are very complementary. If I fuse these three tasks, the performance on this object recognition experiment can be improved to close to 80%. Going back to the stroke problem just now, after fusing the two agent tasks, the performance increased to more than 90%.
Partial supervision 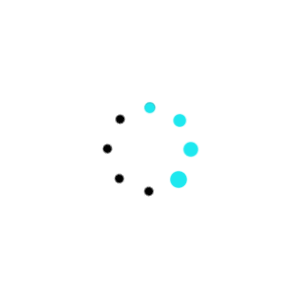
Another example of efficient annotation is partial supervision.
takes organ segmentation as an example. There are currently many different data sets that provide segmentation annotations for different organs. For example, here are five different data sets, one for liver, kidney, spleen, pancreas, etc. It would be very meaningful if we could integrate these five different data sets to expand the amount of data and integrate the annotation information in all data sets.
Our approach is very simple: train a segmentation network and classify each pixel into six categories: liver, pancreas, spleen, left and right kidneys, and background. Therefore, each pixel will output a six-dimensional vector, representing the probability of which category it belongs to (from p0 to p5).
For data with only liver annotation, p1 represents the liver, and the "background" becomes a fusion of the original background and other organs. Because all non-liver pixels are background, the probability of the background becomes five probabilities. Addition, that is, marginal probabilities.Under this condition, we can use marginal probability in the loss function. Through this mechanism, all annotated data can be used for six-category network training, effectively fusing these data together.
At the same time, in this article we also propose an exclusion loss, using a very significant prior knowledge: these organs must not intersect.
For example, going back to the data just labeled with only the liver, I can also calculate p2 (pancreas) to predict the pancreatic area, and the pancreatic area must not intersect with the liver area represented by p1.
Therefore, based on this, a loss function can be designed to make the exclusion of these two areas as small as possible. The results of
training using these two loss functions are as follows. In the experiment, 30 pieces of data were fully annotated. Based on the test based on these data, the obtained segmentation Dice coefficient was 0.87. For training a two-class segmentation network on data with only single organ annotation, the Dice of
is not so high, only 0.85. Through our fusion method, a partially supervised training is performed, utilizing all 688 data in total, and the Dice of our model reaches 0.93.
Therefore, through a very simple idea, we can effectively fuse this data together and improve the performance of segmentation.
Label-free segmentation
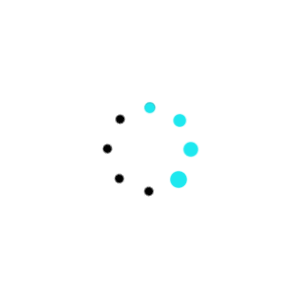
Recently, we have also conducted a relatively "extreme" exploration: CT-based segmentation of new coronavirus pneumonia lesions can also be performed without any labeling.
Our starting point is: not to use segmentation annotation of new coronavirus pneumonia lesions, but to use many CT images without any disease.
uses these normal images and adds some "artificial lesions". If these lesions are similar to COVID-19 lesions, we can learn to segment the lesions. Therefore, we designed an artificial lesion generator whose parameters are manually controlled. Then inject "artificial lesions" into clean images to obtain training samples, and then train a segmentation network. The comparison between
and our method is Anomaly Detection (trained based on normal images to detect whether there are abnormalities).
Anomaly Detection is not very good at segmentation and has low performance. The Dice coefficient in three different COVID-19 data sets is only about 0.3; while our USL method reaches more than 60%, close to 70%. Inf-Net is a semi-supervised method with segmentation performance similar to ours. Of course, the segmentation Dice coefficient obtained by the above method is far from reaching clinical application standards. However, from a research perspective, it is a very interesting exploration.
universal representation learning
universal representation learning aims to learn a universal representation to synthesize heterogeneous tasks, fit multi-domain data, and couple different expressions. It is more in line with the idea of "big tasks, small data".
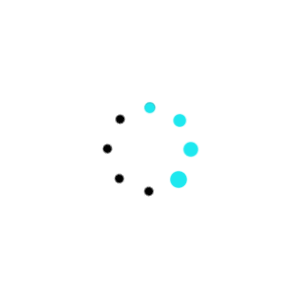
One of our current explorations is also based on the segmentation network. We designed a segmentation network that can be applied to six different segmentation tasks: the input is a CT image, and the required output is liver segmentation; the input is an MRI image, and the required output is prostate segmentation. And so on.
The architecture we adopt is a universal U-Net, but a purple Adapter is introduced for different tasks;
means that each task will utilize these coefficients of the universal network itself, as well as its own adapter part. coefficients, together form a neural network. The advantage of this is that one network can be used to complete the tasks of six networks. The number of parameters of the network is significantly reduced: we use nearly 1% of the number of parameters of the original network and achieve similar segmentation performance as the six networks. Another advantage of
is that common parts of the web can be easily adapted to a new task. If we encounter the seventh task, we only need to fix the common departments and fine-tune the differentiated representation of the seventh task to obtain very competitive segmentation results.
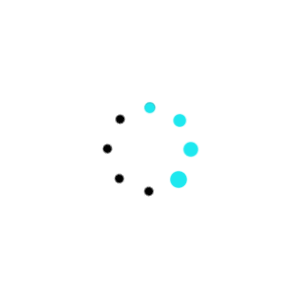
This is another example of a general representation, applied in MR image generation.
From X to Y, we can design a neural network, represented by F, Y=F(X). Usually, we will also design an inverse network: X= F-1(Y), so that we can go back to X from Y.This is a relatively important process because a loop is introduced through which cycle consistency can be defined.
Based on this, we came up with a very simple idea: instead of training two different neural networks, a forward network and an inverse network, we train only one neural network; that is, the two networks are mutually reinforcing Reverse. The training process of
is also relatively simple: take X as input for the first time, and train the network to output Y. The second time, take Y as input and in turn output X. We have achieved very good results in this MR image generation task, improving the signal-to-noise ratio by about 3dB. This effect is quite amazing.
This is also an example of a universal representation, because we use one representation to accomplish two things.
Learning and knowledge integration
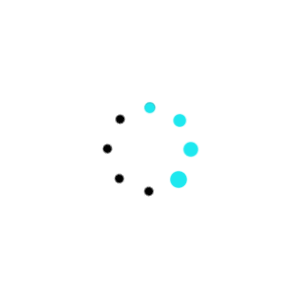
Finally, let’s introduce learning and knowledge integration.
We know that medical imaging has a lot of data, which can be modeled through machine learning (especially deep learning). At the same time, medical imaging has a lot of knowledge, and we can also directly model the knowledge. Therefore, the effect of integrating learning and knowledge is better than machine learning based only on big data.
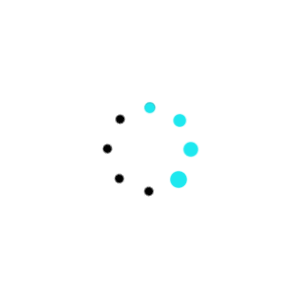
In practice, I often observe that it improves performance. Here are some examples.
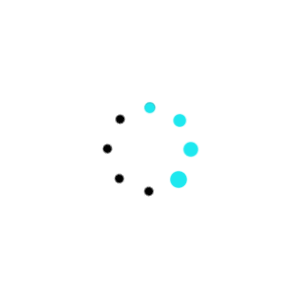
This is an example of automatic diagnosis of chest X-ray.
The general approach is to train a ‘black box’ neural network to directly predict the diagnosis. We looked at a way to use knowledge of anatomical decomposition to improve performance, which we learned from talking to clinicians.
When looking at a chest X-ray for diagnosis, you will observe that the ribs may be blocking the lungs, hindering the diagnosis. Therefore, we designed a decomposition network to divide the X-ray film into three parts: bone projection, lung projection, and other projections, and then input it together with the original image into the neural network for automatic diagnosis of lung diseases. In doing so, much more accurate diagnostic information can be obtained from the lung projection in the middle. Experimental results show that among 14 types of diseases, 11 types of diseases have better diagnosis and prediction, and most of these 11 types of diseases are directly related to the lungs.
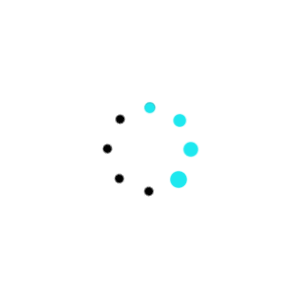
The second example is unpaired artifact removal: give the neural network a picture with artifacts and eliminate the artifacts through learning.
This is the design of our network. The modules are built like Lego. A lot of knowledge is used in the construction process. In the end, the network can also successfully separate artifacts. The neural network built using knowledge has much better performance than the general black box method.
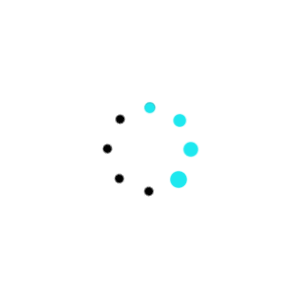
Another example, the intra-layer accuracy of medical imaging is relatively high, but the inter-layer accuracy is not very high, and a lot of inter-layer information will be blurred.
If it is a regular CT spine image with insufficient inter-slice accuracy, even the bones will not be visible clearly after rendering.
We have recently tried to perform inter-layer interpolation work, which can effectively restore the information between layers and is more conducive to diagnosis (the effect is as shown above). The algorithm itself uses specific knowledge of image accuracy, so we also present it as an example of "learning and knowledge fusion". Please refer to the published article for specific algorithm details.
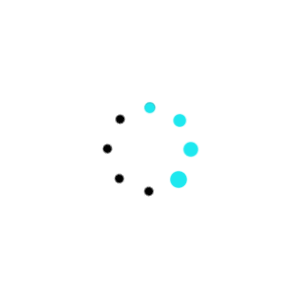
To summarize, we analyzed the seven major characteristics of medical imaging and the corresponding algorithm trends we proposed around these seven major characteristics.
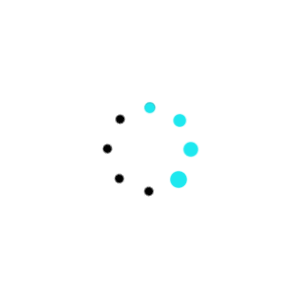
Recently, we also wrote a review article, which was also accepted by the Proceedings of IEEE.
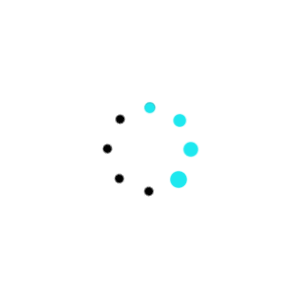
Finally, let’s introduce MONAI.
MONAI is a completely open source community that can provide researchers with deep neural network resources for medical image analysis. A dedicated team builds and tests this software, so the reliability of the software is very high.
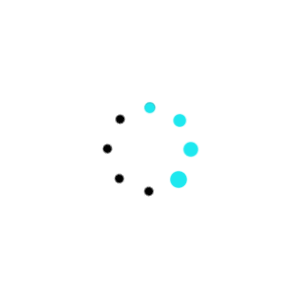
I am also a consultant for the MONAI project. We will put forward many of these requirements and hope that everyone can use MONAI. leifeng.com