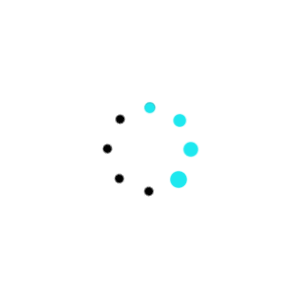
Author | Li Mei, Liu Bingyi
Editor | Chen Caixian
Following the cooperation of 100 Stanford authors to publish the "Foundation Model" research review and the Zhiyuan collection of 100 authors to publish the big model research review (it was later revealed to be "turned off"), recently, another paper co-authored by more than 100 authors appeared in the AI circle!
This paper ("Beyond the Imitation Game: Quantifying and Extrapolating the Capabilities of Language Models") was published by Google and gathered 442 authors!
In the PDF document of the paper, the author list occupies a whole page:
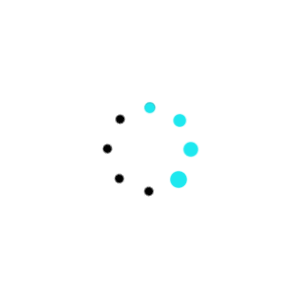
Thesis address: https://arxiv.org/pdf/2206.04615.pdf
GitHub: https://github.com/Google/BIG-bench
Type all names on the same page. It is quite a test of your vision if you want to find the name of a certain author.
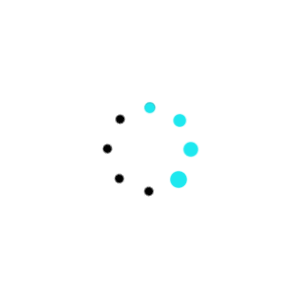
is huge in scale, and one of the authors William Fedus sighs that this is really It takes an army.
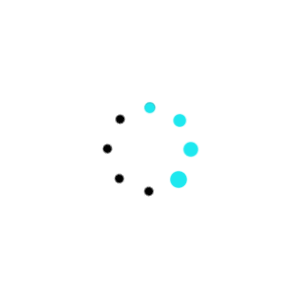
articles total 100 pages, and the references start from 51 pages, accounting for half of the space.
Because there are too many participants in the study, it is hard to tell who has made greater contributions in one sentence or two sentences. So I just set up a chapter in the article to explain the efforts of all the public.
is not much space, only 15 pages. The core contributions listed by
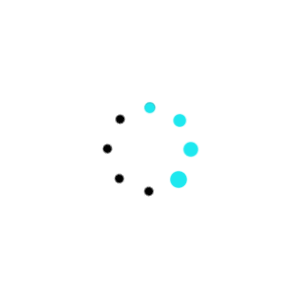
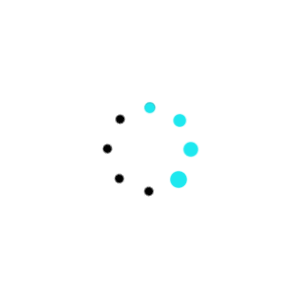
include Guy Gur-Ari, Ethan Dyer, Ambrose Slone, etc. They perform the new benchmark for large language model BIG-bench github code infrastructure and documentation.
and Review, which provides tasks...
However, these specially mentioned core contributors are not at the top of the author column of the article, because this article does not distinguish the first author, and the order of the author column is arranged in alphabetical order of .
The response on Twitter is pretty good, and some readers say that the work "seems to be a gold mine, extraordinary cooperation 👏🏻".
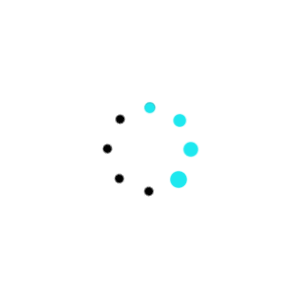
also commented: "I appreciate the organizers' leadership in promoting the completion of this work! The exciting large-scale cooperation model will benefit the entire community."
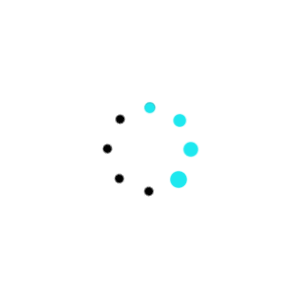
(curiously Google has gathered so many people to co-author. Have you done "paper checking"? We dare not say it, and we dare not ask)
So, what exactly does this work talk about?
1 New benchmark for big model: BIG-Bench
It is understood that this article is Google's publication of BIG-Bench paper and GitHub.
BIG bench consists of 204 tasks, and the task topics involve issues in the fields of linguistics, child development, mathematics, common sense reasoning, biology, physics, social bias, software development, etc.
In the PaLM model architecture of Jeff Dean et al., the researchers conducted multitask tests on BIG-Bench’s large-model-specific benchmark.
The study lasted for 2 years , and there were many changes in the work unit among the hundreds of people.
Google launched a new benchmark for big models because its performance has been improved as the language model continues to expand in size, and some new performances may have potential transformational impacts, but have not yet been clarified. To evaluate the performance and limitations of existing language models, the authors’ team specially introduced the benchmark BIG-bench.
Beyond the Imitation Game Benchmark (BIG-bench) GitHub repository includes:
More than 204 language tasks. As with the BIG-bench review criteria, the benchmark task covers different topics and languages and is something that cannot be completely solved by the current model.
BIG-bench Lite: A small, representative subset of tasks that perform faster evaluation than on the entire benchmark.
Code to implement the benchmark API: Support task evaluation on publicly available models and implement lightweight creation of new tasks.
detailed evaluation results of dense and sparse language models with scales across six orders of magnitude, as well as baseline results established by human assessors.
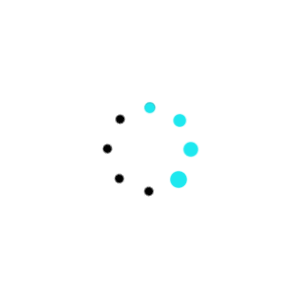
BIG-bench supports two types of tasks: JSON and programming tasks, of which about 80% of the benchmark tasks are JSON tasks.
JSON task is defined by a JSON file that contains a list of examples composed of inputs and targets. Performance is evaluated by using standard metrics such as ROUGE or based on the probability assigned by the model (such as answering multiple choice questions). The example-based JSON task specification also allows for simple, low-sample evaluation.
Another about 20% of the benchmark tasks are programmatic, written in Python, can interact directly with the model in multiple rounds of queries, and can measure performance using custom metrics. Using model objects to call programming tasks, you can query the model using the following methods:
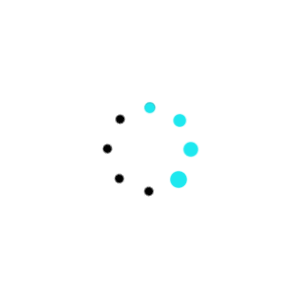
2 BIG-bench evaluation found
The author team evaluated the capabilities of multiple language models on BIG-bench, with model sizes ranging from millions to hundreds of billions of parameters, including the GPT model of OpenAI, Google's internal intensive transformer architecture, and the performance of Switch-style sparse transformer, etc.
Although language models have good performance due to their large scale, they still perform poorly on BIG-bench compared to humans.
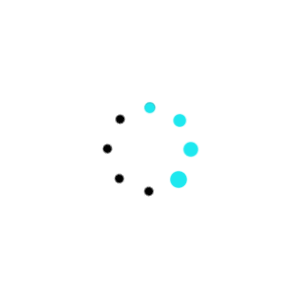
They also evaluated Google's own PaLM model, which showed that its performance beat other models before PaLM (dog heads), and although PaLM is still below the best human raters (dark blue dotted line in the picture below), it has surpassed the average human raters on the BIG-bench Lite partition (blue dotted line in the picture below).
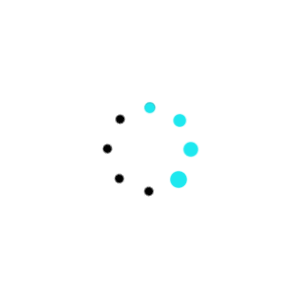
In some tasks, the performance of the language model will steadily improve with the increase of scale; in other tasks, the language model will suddenly produce breakthrough performance on a specific scale.
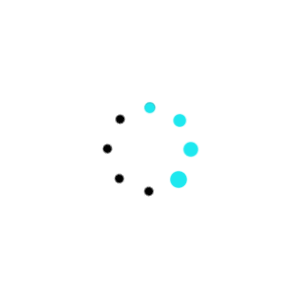
After evaluation, they also found that as the model scales, their social biases are becoming more and more prominent. One possible explanation for this is that larger models do a better job of matching the biases in their training sets. However, when the context clearly shows that bias is not desirable, bias decreases as it grows. This result of
emphasizes the importance of research, engineering and policy efforts to address the fairness of machine learning systems.
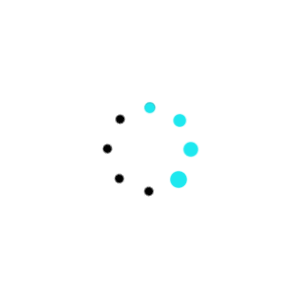
To solve the problem of social bias in the model, the authors' team gave three findings: 1) In the context of widespread or ambiguous, bias usually increases with the scale; 2) In narrow and clear contexts, bias decreases with the scale; 3) bias can be guided by selecting appropriate prompts.
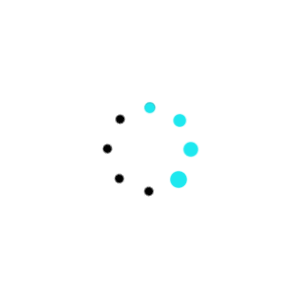
Figure Note: For contexts with clear or positive prompts, bias may decrease with changes in scale, or be more stable,
They also found that the model performed better on English tasks than non-English tasks, and performed especially poorly on tasks involving low-resource languages. In some cases, the performance of low-resource language tasks does not improve with the increase in the size of the model, while the performance of corresponding English tasks will improve with the increase in the size.
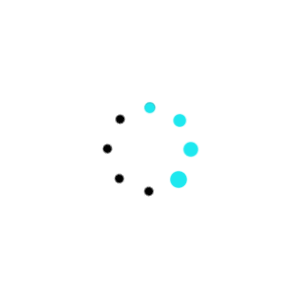
Overall, sparse models perform as well as intensive models that use 2x more inference costs, and their calibration is as good as intensive models that use about 10x more inference calculations.
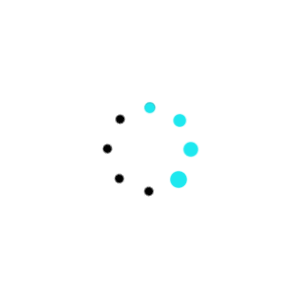
When manually checking the model output, the team found that the model started to generate movie titles after a certain scale, and at a larger scale it began to recognize the semantics of the emoji , and in some cases the correct answer was output at the maximum scale. A representative example is shown in the figure below:
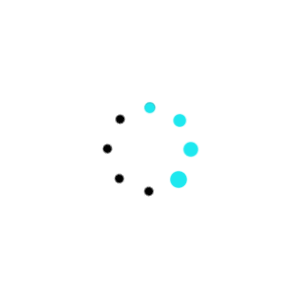
Figure Note: According to the precise task indicators, the performance in emoji_movie recognition will appear sudden or gradual.
In addition, they found that the programming ability of the model is very subjective. Even quantified through specific tasks, the capabilities of language models and trajectories across scales are much more subjective than we think.
Then think about the "Is AI a personality" that has been in full swing these two days... What do you think of
?
Reference link:
https://arxiv.org/pdf/2206.04615.pdf
https://github.com/google/BIG-bench
https://twitter.com/jaschasd/status/1535055886913220608/retweets/with_comments
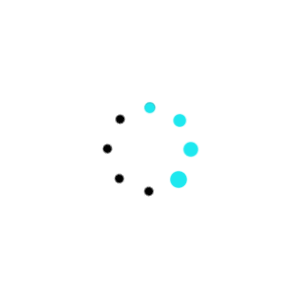